Learning Analytics and Educational Management
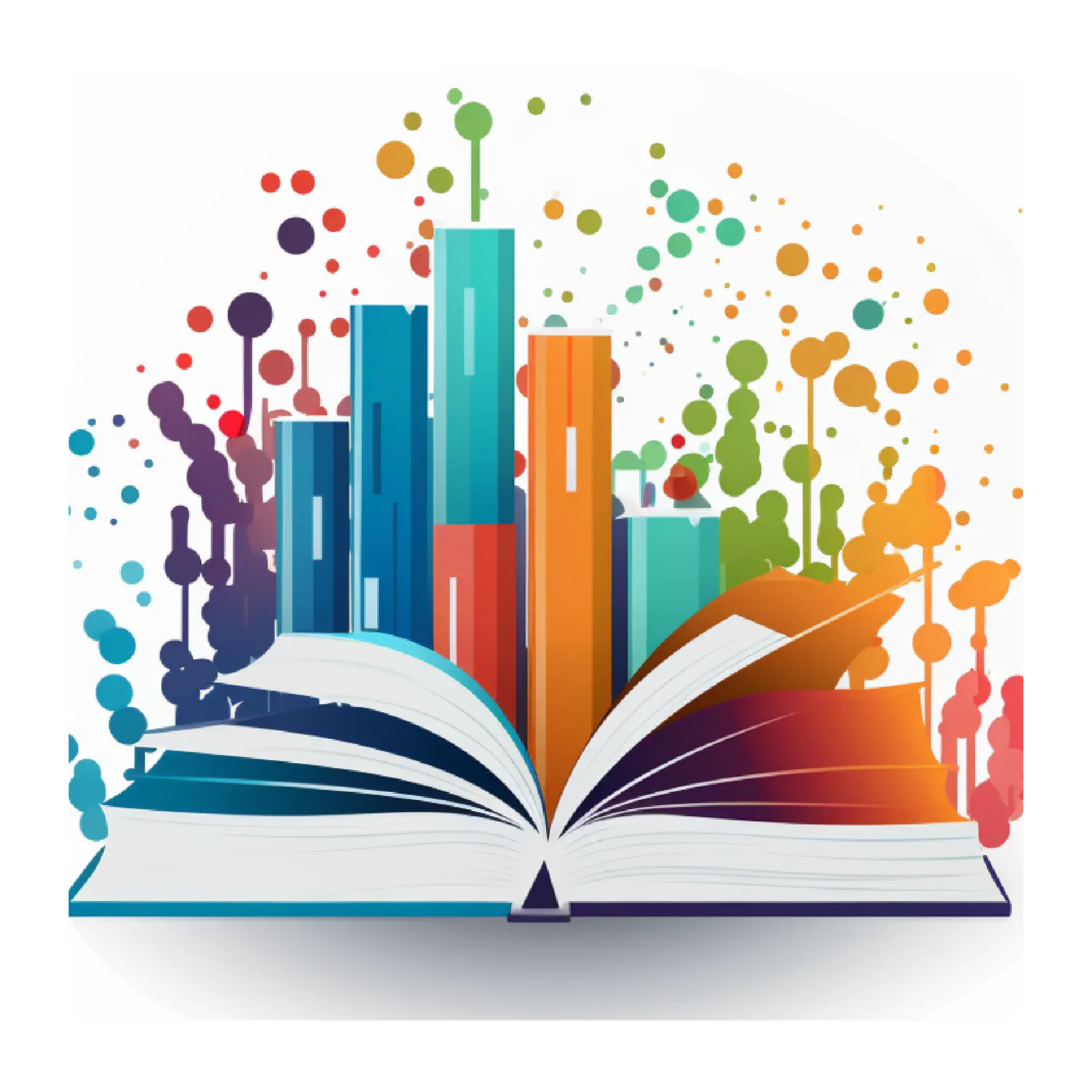
Learning Analytics is an interdisciplinary field that integrates data analysis, computer science, and educational psychology to improve learning and educational environments. By collecting, analyzing, and interpreting data about learners and their contexts, it offers insights for personalizing education, identifying students in need of additional support, and enhancing educational outcomes. In educational management, it is important for providing significant data for decisions about resources and teaching strategies, helping to identify trends and future needs. Its integration represents a significant shift in education, making it more data-driven, responsive, and student-centered.
About the Area Coordinators
Prof. Sergio Antônio Andrade de Freitas
More About the Coordinator
Profa. Cristiane Soares Ramos
More About the Coordinator
Research Team
Previous Researchers
Master’s degree
- Éber Junio Borges Moreira,
Scheduling of Academic Resources in Higher Education Institutions: A Case Study at the University of Brasília
. Master's Thesis in Applied Computing, University of Brasília (Brazil), 2024
Advisor(s):
Sergio Freitas
. Tags:
Learning Analytics,
Machine Learning
.The scheduling of academic resources in higher education institutions is a highly complex problem, involving the allocation of classes, professors, time slots, and physical spaces to meet multiple institutional constraints and preferences. This paper presents the SARA model (Academic Resource Scheduling System), designed to offer flexibility and adaptability to the specific needs of higher education institutions. The research was conducted in two main stages: a systematic literature review and the proposal of the SARA model. The systematic review enabled the mapping of widely used strategies, algorithms, and constraints in the literature, providing theoretical foundations for the model's development. The SARA proposal integrates features such as customization, integration with academic systems, and the application of optimization algorithms, promoting a comprehensive and adaptable approach to scheduling in university environments. The model was validated through a case study at the University of Brasília (UnB), in a large-scale and highly complex environment. Preliminary results demonstrated the model's feasibility and effectiveness in meeting the institution's specific demands, highlighting its potential applicability in various institutional contexts. The research contributes to theoretical and practical advancements in academic management, presenting a solution that combines methodological rigor with practical applicability.
- Edmilson Cosme da Silva,
Prediction of academic dropout in higher education - the case of face-to-face undergraduate courses at the University of Brasília
. Master's Thesis in Applied Computing, University of Brasília (Brazil), 2023
Advisor(s):
Sergio Freitas
. Tags:
Learning Analytics,
Machine Learning
.Researchers have been investigating attrition in higher education, identifying two categories - students who leave the university and those who abandon higher education altogether, negatively affecting institutions, students, and society. Since 1995, in Brazil, the creation of ANDIFES has led to more frequent studies on graduation, retention, and attrition in Brazilian universities, focusing on institutional attrition, characterized by a student's departure from their original course. The University of Brasília (UnB) has implemented strategies to increase student retention in undergraduate courses. This work aimed to develop and test an analysis model to predict attrition in face-to-face courses, through a Systematic Literature Review to identify impact factors and define indicators extracted from UnB's academic systems. The model, named MAGRA, combines indicators with machine learning algorithms to identify students at risk of dropping out. Tests conducted at the Faculdade do Gama (FGA) and UnB indicated that enrollment frequency in subjects could predict completion difficulties. The research suggests that to improve the early identification of at-risk students, adjustments in feedback mechanisms, the inclusion of new systems, improvement in data quality, and adjustments in algorithm parameters are necessary.
Bachelor’s Thesis
- Allan Nobre, Using Learning Analytics and Educational Data Mining to Support Pedagogical Decisions During TBL Methodology Application . Senior Project (Bachelor of Software Engineering) - University of Brasília (Brazil), 2023 Advisor(s): Cristiane Ramos . Tags: Learning Analytics, Education .
- Amanda Emilly Muniz de Menezes,
Identifying Factors Affecting Dropout in Higher Education
. Senior Project (Bachelor of Software Engineering) - University of Brasília (Brazil), 2022
Advisor(s):
Cristiane Ramos,
Sergio Freitas
. Tags:
Education,
Learning Analytics
.Identifying factors that influence academic dropout is crucial for coordinators to take preventive actions against student desertion. This thesis aims to explore the current state of research in the field, seeking to understand the main factors associated with academic dropout and how they can be applied in predictive models of dropout. A quantitative, exploratory, and explanatory applied research was conducted, using case study techniques and bibliographic research. The systematic literature review indicated that dropout could be predicted by academic, demographic, and learning factors. Using these factors, predictive models were developed with the help of machine learning algorithms, whose predictions proved sensitive to changes in course flows. It concludes that academic, demographic, and learning factors are effective in predicting dropout, with the selection and application method of these factors being crucial for achieving accurate predictive results. Moreover, the application of academic factors in predictive models requires consideration of the specific context of the analyzed data.
- Letícia Karla Soares Rodrigues de Araújo,
Identifying Factors Affecting Dropout in Higher Education
. Senior Project (Bachelor of Software Engineering) - University of Brasília (Brazil), 2022
Advisor(s):
Cristiane Ramos,
Sergio Freitas
. Tags:
Education,
Learning Analytics
.Identifying factors that influence academic dropout is crucial for coordinators to take preventive actions against student desertion. This thesis aims to explore the current state of research in the field, seeking to understand the main factors associated with academic dropout and how they can be applied in predictive models of dropout. A quantitative, exploratory, and explanatory applied research was conducted, using case study techniques and bibliographic research. The systematic literature review indicated that dropout could be predicted by academic, demographic, and learning factors. Using these factors, predictive models were developed with the help of machine learning algorithms, whose predictions proved sensitive to changes in course flows. It concludes that academic, demographic, and learning factors are effective in predicting dropout, with the selection and application method of these factors being crucial for achieving accurate predictive results. Moreover, the application of academic factors in predictive models requires consideration of the specific context of the analyzed data.
- Yeltsin Suares Gama, Learning Support System. . Senior Project (Bachelor of Software Engineering) - University of Brasília (Brazil), 2017 Advisor(s): Sergio Freitas . Tags: Learning Analytics, Artificial Intelligence .
Current Projects
- A new approach applied to the University Course Timetable Problem
Éber Júnio Borges Moreira, Start: 2023, status: ongoing.
Work focused on optimizing university course scheduling, applying techniques to improve efficiency and effectiveness in academic timetable management. This work aims to solve common challenges in timetable management, providing a more adaptable and convenient solution for higher education institutions. UnB is used as a case study.
Publications and Productions
Publications (17)
- Éber Júnio Borges Moreira,Sergio Antônio Andrade Freitas, A CP-SAT Approach for Academic Resource Timetabling in Higher Education Institutions: A Case Study at a Major Public University , in IEEE International Conference on IT in Higher Education and Training (ITHET 2024) , pTo appear, 2024 . Tags: Machine Learning, Learning Analytics .
- Sergio Antônio Andrade Freitas,Cristiane S. Ramos,Eduardo Bessa Pereira da Silva,Marcia Renata Mortari,Dianne Magalhaes Viana, Implementing Neuroscientific Principles in Gamified Software Engineering Courses , in Frontiers in Education 2024 , pTo appear, 2024 . Tags: Active Learning, Learning Analytics, Gamification .
- RAMOS, C. S.,VIANNA, D. M.,BESSA, E.,MORTARI, M. R.,FREITAS, S. A. A., Indicadores de aprendizagem , Biblioteca Central da Universidade de Brasília, 2023 . Tags: Learning Analytics, Active Learning .
- SILVA, E. C.,FREITAS, S. A. A.,RAMOS, C. S.,MENEZES, A. E. M.,ARAUJO, L. K. S. R., A systematic review of the factors that impact the prediction of retention and dropout in higher education , in 56th Hawaii International Conference on System Science , 2023 . Tags: Learning Analytics, Machine Learning .
- Éber Junio Borges Moreira,
Scheduling of Academic Resources in Higher Education Institutions: A Case Study at the University of Brasília
. Master's Thesis in Applied Computing, University of Brasília (Brazil), 2024
Advisor(s):
Sergio Freitas
. Tags:
Learning Analytics,
Machine Learning
.The scheduling of academic resources in higher education institutions is a highly complex problem, involving the allocation of classes, professors, time slots, and physical spaces to meet multiple institutional constraints and preferences. This paper presents the SARA model (Academic Resource Scheduling System), designed to offer flexibility and adaptability to the specific needs of higher education institutions. The research was conducted in two main stages: a systematic literature review and the proposal of the SARA model. The systematic review enabled the mapping of widely used strategies, algorithms, and constraints in the literature, providing theoretical foundations for the model's development. The SARA proposal integrates features such as customization, integration with academic systems, and the application of optimization algorithms, promoting a comprehensive and adaptable approach to scheduling in university environments. The model was validated through a case study at the University of Brasília (UnB), in a large-scale and highly complex environment. Preliminary results demonstrated the model's feasibility and effectiveness in meeting the institution's specific demands, highlighting its potential applicability in various institutional contexts. The research contributes to theoretical and practical advancements in academic management, presenting a solution that combines methodological rigor with practical applicability.
- Edmilson Cosme da Silva,
Prediction of academic dropout in higher education - the case of face-to-face undergraduate courses at the University of Brasília
. Master's Thesis in Applied Computing, University of Brasília (Brazil), 2023
Advisor(s):
Sergio Freitas
. Tags:
Learning Analytics,
Machine Learning
.Researchers have been investigating attrition in higher education, identifying two categories - students who leave the university and those who abandon higher education altogether, negatively affecting institutions, students, and society. Since 1995, in Brazil, the creation of ANDIFES has led to more frequent studies on graduation, retention, and attrition in Brazilian universities, focusing on institutional attrition, characterized by a student's departure from their original course. The University of Brasília (UnB) has implemented strategies to increase student retention in undergraduate courses. This work aimed to develop and test an analysis model to predict attrition in face-to-face courses, through a Systematic Literature Review to identify impact factors and define indicators extracted from UnB's academic systems. The model, named MAGRA, combines indicators with machine learning algorithms to identify students at risk of dropping out. Tests conducted at the Faculdade do Gama (FGA) and UnB indicated that enrollment frequency in subjects could predict completion difficulties. The research suggests that to improve the early identification of at-risk students, adjustments in feedback mechanisms, the inclusion of new systems, improvement in data quality, and adjustments in algorithm parameters are necessary.
- Allan Nobre, Using Learning Analytics and Educational Data Mining to Support Pedagogical Decisions During TBL Methodology Application . Senior Project (Bachelor of Software Engineering) - University of Brasília (Brazil), 2023 Advisor(s): Cristiane Ramos . Tags: Learning Analytics, Education .
- CABELLO, A. F.,ARRUDA, J. A.,IMBROISI, D.,FERREIRA, G. V.,FREITAS, SERGIO A. A., Strategic Choice in Selective Processes: analyses of the alteration of initial option of candidates in SISUnB, and its effects on dropout , REVISTA COM CENSO ESTUDOS EDUCACIONAIS DO DISTRITO FEDERAL , 9(41-49), 2022 . Tags: Learning Analytics .
- Amanda Emilly Muniz de Menezes,
Identifying Factors Affecting Dropout in Higher Education
. Senior Project (Bachelor of Software Engineering) - University of Brasília (Brazil), 2022
Advisor(s):
Cristiane Ramos,
Sergio Freitas
. Tags:
Education,
Learning Analytics
.Identifying factors that influence academic dropout is crucial for coordinators to take preventive actions against student desertion. This thesis aims to explore the current state of research in the field, seeking to understand the main factors associated with academic dropout and how they can be applied in predictive models of dropout. A quantitative, exploratory, and explanatory applied research was conducted, using case study techniques and bibliographic research. The systematic literature review indicated that dropout could be predicted by academic, demographic, and learning factors. Using these factors, predictive models were developed with the help of machine learning algorithms, whose predictions proved sensitive to changes in course flows. It concludes that academic, demographic, and learning factors are effective in predicting dropout, with the selection and application method of these factors being crucial for achieving accurate predictive results. Moreover, the application of academic factors in predictive models requires consideration of the specific context of the analyzed data.
- Letícia Karla Soares Rodrigues de Araújo,
Identifying Factors Affecting Dropout in Higher Education
. Senior Project (Bachelor of Software Engineering) - University of Brasília (Brazil), 2022
Advisor(s):
Cristiane Ramos,
Sergio Freitas
. Tags:
Education,
Learning Analytics
.Identifying factors that influence academic dropout is crucial for coordinators to take preventive actions against student desertion. This thesis aims to explore the current state of research in the field, seeking to understand the main factors associated with academic dropout and how they can be applied in predictive models of dropout. A quantitative, exploratory, and explanatory applied research was conducted, using case study techniques and bibliographic research. The systematic literature review indicated that dropout could be predicted by academic, demographic, and learning factors. Using these factors, predictive models were developed with the help of machine learning algorithms, whose predictions proved sensitive to changes in course flows. It concludes that academic, demographic, and learning factors are effective in predicting dropout, with the selection and application method of these factors being crucial for achieving accurate predictive results. Moreover, the application of academic factors in predictive models requires consideration of the specific context of the analyzed data.
- CABELLO, A. F.,FERREIRA, G. V.,IMBROISI, D.,ARRUDA, J. A.,FREITAS, S. A. A.,ALVAREZ, G. A., Forms of admission in a comparative perspective: why does SISU increase dropout? The case of UNB , AVALIAÇÃO: REVISTA DA AVALIAÇÃO DA EDUCAÇÃO SUPERIOR , 26(446-460), 2021 . DOI: 10.1590/s1414-40772021000200006 . Tags: Learning Analytics, Education .
- CABELLO, A. F.,FERREIRA, G. V.,IMBROISI, D.,ARRUDA, J. A.,FREITAS, S. A. A.,ALVAREZ, G. A., Forms of Admission in Comparative Perspective: Sisu at UnB , in XIX Colóquio Internacional de Gestão Universitária , 2019 . Tags: Learning Analytics .
- FREITAS, S. A. A.,CANEDO, E. D.,JESUS, D. A., Calculating Similarity of Curriculum Lattes , IEEE Latin America Transactions , 16(1758), 2018 . DOI: 10.1109/tla.2018.8444396 . Tags: Learning Analytics .
- COSTA OLIVEIRA, EDGARD,ALVES DE JESUS, DIONLAN,FREITAS, S. A. A.,DIAS CANEDO, EDNA, Similarities Building a Network between Researchers based on the Curriculum Lattes Platform , in 20th International Conference on Enterprise Information Systems , 2018 . DOI: 10.5220/0006664102030214 . Tags: Learning Analytics .
- Yeltsin Suares Gama, Learning Support System. . Senior Project (Bachelor of Software Engineering) - University of Brasília (Brazil), 2017 Advisor(s): Sergio Freitas . Tags: Learning Analytics, Artificial Intelligence .
- FREITAS, S. A. A.,SILVA, RITA C.,CANEDO, EDNA D.,LUCENA, TIAGO FRANKLIN R., A tool for students' grouping in classroom , in 2016 IEEE Frontiers in Education Conference (FIE) , 2016 . DOI: 10.1109/FIE.2016.7757708 . Tags: Learning Analytics .
- LOZZI, S.,DINIZ, F. B.,ALBURQUERQUE, P.,REZENDE, M.,FREITAS, S. A. A.,COSTA, C., Influence of Affirmative Action on the Academic Performance of Undergraduate Students from a Brazilian Public University , in 5th International Conference of Education, Research and Innovations, 2012, Madrid - Spain , pp. 3017-3022, 2012 . Tags: Learning Analytics .
Apps and programs
- FREITAS, S. A. A. Analyzer of quality indicators of Brazilian higher education. UnB, 2020.
- FREITAS, S. A. A. Collaborative editor for pedagogical course projects - UnB version. 2019.
- FREITAS, S. A. A. MCE - Curriculum Matrix Editor. UFES, 2005.
- FREITAS, S. A. A. Course Pedagogical Project Generator. UFES, 2005.
- FREITAS, S. A. A. Academic Management System for Postgraduate Courses at the Federal University of Espírito Santo (UFES). UFES, 2002.
Contact & Collaboration
- Email for information and contact with the team: sergiofreitas@unb.br or cristanesramos@unb.br.